Driving Customer Engagement Through Data-Driven Collaboration
Achieving next-level customer engagement requires both innovative data strategies and seamless teamwork. At our MarTech and Data Solutions Center of Excellence, our Business Intelligence Strategist and Senior MarTech Director collaborated to help a major streaming client redefine customer retention through data-driven insights.
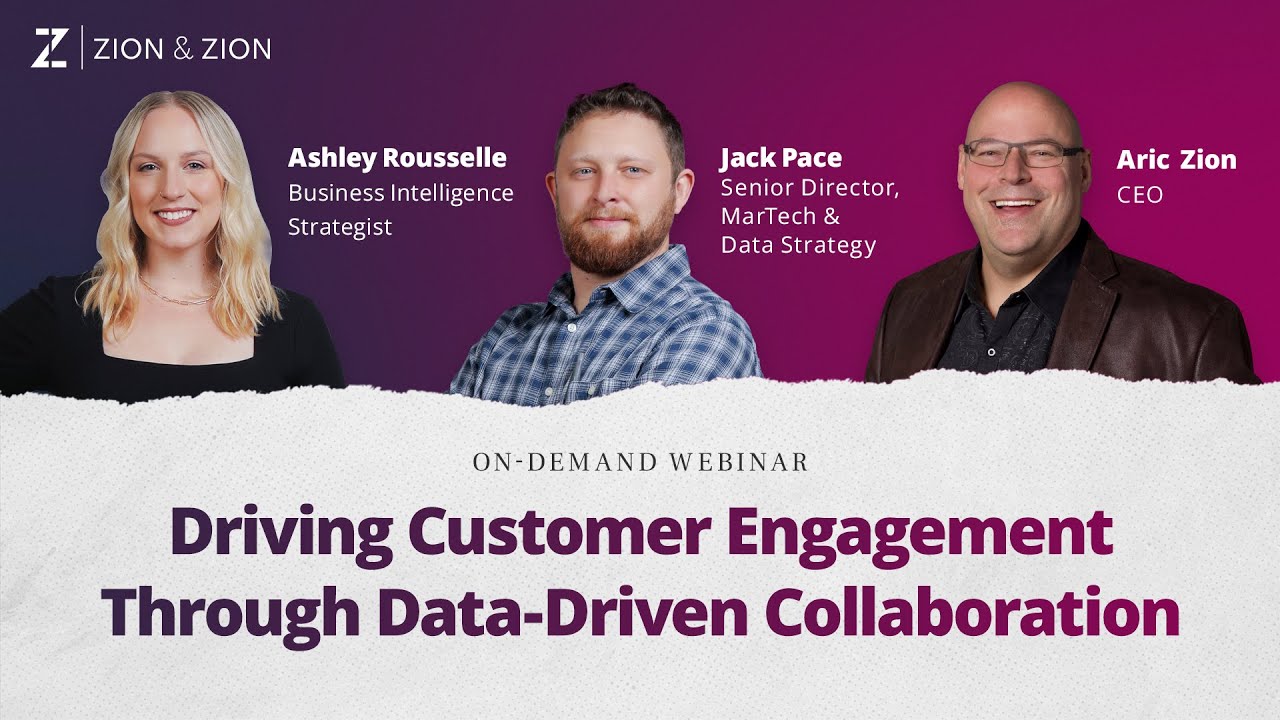
Key Insights
- Our team combined expertise in data strategy and marketing technology to solve complex customer engagement challenges.
- Leveraging advanced data insights, we quickly optimized retention strategies for a leading streaming client.
- Seamless teamwork across roles enabled us to create measurable, data-driven outcomes.
Aric
Well, welcome everyone. We have yet another in our series of collaboration-oriented webinars. I think this is a really, really interesting one.
First, we have with us two people from our MarTech and Data Strategy Data Solutions Center of Excellence, Ashley Roussel, a business intelligence and data visualization strategist, and Jack Pace, Senior Director of MarTech and Data Solutions. And this is a really, really interesting challenge that we had with a client that they and others on the team had to collaborate to solve. We’ll tell you this is a fairly high-profile streaming media client, but I’m actually going to turn it over to them to talk through the problem that had to be solved and then the deep levels of collaboration and technology problem resolution that went in to solve it.
So which of you would like to take that kind of a description of the problem that the Center of Excellence had to solve?
Ashley
Yeah, I can take that one. So this client uses a lifecycle marketing tool for their email engagement and sending out their different emails. So the problem that we have is that they have very different campaign types that they’re sending out.
And with these different campaign types, they’re being sent to a very unique set of users, each one of them. So with that, we really need to be able to tell, did these users that engaged with email engage beyond that email to that desired action? So with that, within the lifecycle marketing tool, we can really tell if they open the email, if they click the email.
But to determine the success of these campaigns, we needed to get beyond that email interaction and ask the question, did they take the desired action after that email interaction? So with that, we really had to pull in a lot of different data sources than what we would find in the lifecycle marketing tool to be able to answer these different questions for the client and really determine if their email campaigns were successful or not.
Aric
And when you say lifecycle marketing tool, obviously they all track email opens and clicks, et cetera. You can put conversion metrics or conversion actions tracked on landing pages, et cetera. But this was far more complicated than did somebody take an action on a landing page as a result of viewing or clicking this email?
Jack, do you want to talk about that? I think you had an example or two of what those downstream conversion actions we were looking for and why it wasn’t quite as simple as just to tag something and see if the conversion occurred.
Jack
Yeah. So there is different campaign types from win-back, engagement and retention, churn mitigation. And so all of these different campaign types had their own unique set of conversion success metrics.
And like you said, a simple tag would not suffice just to track, okay, how many of this particular event occurred based off this campaign type. So looking at churn mitigation, we needed to look at how many users were pausing their subscription at the end of a season for win-back. It was how many users are signing back up, not just signing back up, but also converting to paid after a period of time from a pre-trial or a promo.
And then on engagement and retention, that was the most kind of unique one as we wanted to measure from those campaigns, how many users are engaging with content downstream. So could have been a metric of they watched something after getting the email that could have been tagged, but it was deeper than that. It’s how much content did they consume from a minute’s perspective?
How many different episodes did they watch after that engagement? And then also bringing this stuff all into the warehouse, writing queries, joining all this data together allowed us to have a flexible attribution window setting so that if we wanted to say, okay, for this campaign, the conversion window is 24 hours. For another campaign, it’s seven days or 30 days.
So we were able to uniquely set up these queries to measure the success based off of the criteria for that conversion event, as well as that attribution window to attribute those downstream conversions back to that engagement.
Aric
I’m really curious. So I know that we have multiple team members in the Market Data Solutions Center of Excellence assigned to this account of ours, multiple functions, data science, data engineering, analytics strategists, et cetera. So what were some of the collaborations that had to happen, especially since that’s the focus of this webinar?
It wasn’t just one person. What were some of the different roles involved in building the infrastructure to make everything you guys did a success?
Jack
Yeah, this took a small army to really put together. And it wasn’t just MarTech and Data Solutions. So from the MarTech and Data Solutions side, we had, of course, Ashley and myself, but then we also had our senior data engineer who was setting up a lot of the pipelines to bring in all of the raw log level data across these data sources.
We had a data solutions architect who came in to help from a solutions perspective, what is this going to look like for that data engineer or senior data engineer to build? We leveraged our data scientists just for input, especially around attribution and what would be the correct attribution window for some of these campaigns. And then we also brought in a senior expert on our lifecycle marketing team as well to help really from a client perspective, what should they be looking at?
What is important? Because we wanted the whole kitchen sink at it. And this individual helped us dial it in to really get to the core of what we really needed to answer the questions that they were asking, but then also help the client refine the questions to say, hey, maybe that’s not the right question to be asking.
But your question over here is going to yield the insights that are going to drive this kind of data driven optimization to their different campaigns.
Aric
Got it. So, I mean, definitely really exciting. Can you talk about some of the, what does it take to run a center of excellence like this with all these different functions and all these different parts?
Because I mean, for people listening to this, they probably aren’t aware that in most orgs, all of those different functions, many of them sit in very different departments. In the center of excellence, they’re really organized in a matrix collaborative approach. What does it take though, when somebody says, okay, you have all these resources in the center of excellence, get them to work together, to collaborate, to understand each other’s language, et cetera.
Talk about that a little bit.
Jack
Yeah, that’s a good question. So it really starts at the program level. So we established this data program where early on in the year, we say, here’s our three main objectives that align very closely to the client or the business that we’re working with.
And from those, the data program oversees several different projects, all marching to those three, five objectives. And everyone has a role within that program on each of those projects being either quick, rapid intervention to come in to help unlock a particular problem that they’re facing on a project or lead the project separately. So it really comes to that program level management where you have that north star that you’re all working towards and those projects that all kind of connect with each other to fulfill the objectives that are set for that data program.
And again, big alignment with the organizational objectives because that’s what helps establish the projects that the data program manages within it.
Aric
Got it. And Ashley, I know this wasn’t originally one of the questions that we were going to ask during this because unsurprisingly, of course, we did do a little prep for this, but I’m going to throw you an ad hoc one. So you’ve been part of our agency, part of the Center of Excellence for a couple of years now.
What is your journey through these different functions, right? Because you haven’t always had the same role in that Center of Excellence. What has that been like for you?
And why don’t you talk about that a little bit?
Ashley
Yeah, absolutely. So I started as a senior analytics strategist where I was working really heavily in the CDP space where we’re really working on strategies, implementations, and then kind of moved over more to the data visualization side. So with that, I’ve been able to work with a lot of different teams internally and now even more so externally as well.
So with that, we really understand how to work together as a team. We understand this is what I’m going to do. This is what you’re going to do.
This is how we’re going to help each other. So I think throughout just moving different roles within the agency and getting to collaborate with different team members, we really figure out how we all click together. And it makes it a lot easier to collaborate on big projects like this when we have our defined roles and we clearly understand like this is what I do.
This is what you do. And of course, there’s overlap on both of those. And we all collaborate and we all have brainstorming sessions on how we think the best way to handle different approaches are.
So I think moving roles within the agency has really helped with that collaboration side. And then really from the external side as well of how do we collaborate with our clients as well and make sure that they have a say, especially when it comes to data visualization and you’re handing off something that you built to somebody from another team, you want to make sure that it is built to their preferences and that it makes sense for them because they’re the ones who are going to be in it day to day.
What might make sense most for me is maybe not how they read and perceive data on their side. So the collaboration externally is really big because especially when it comes to data visualization and dashboards, there’s a huge risk that you hand off a dashboard to an external team and the adoption rate maybe isn’t very high because you didn’t build it specific to that audience. So that collaboration on this specific project was huge.
Aric
And I know also speaking of more of that collaboration on this project and others, you’ve worked with our UX team as well on structuring the data visualizations from a user experience point of view as well. So that’s an interesting collaboration as well. Can you talk a little bit about that?
Ashley
Yeah, absolutely. So when it comes to dashboards, they’re of course very design heavy. So it only makes sense that we work with UX team and we figure out what makes sense from a design perspective and how people read different dashboards, websites.
It’s all very important to consider. When you’re considering design, you want to make sure that your colors make sense, that for this specific client, if they have a brand color that might be red, green, you want to be careful when you use those different colorings within the dashboard because red can mean alarming, green means good. But when you’re talking about different just brand colors in general, you have to be careful what colors you use and how people perceive those specific colors.
So even details down to that, I mean, details down to the placement of filters, if there’s too many words in the titles, our UX team was really able to guide us on that’s too many words, that’s not enough words, the placement of this is a little confusing. So I think that collaboration was also huge because from a design perspective, in dashboards, we’re really looking at the data presented and not as much as how the data is perceived. So that UX collaboration was really big within these dashboards.
Aric
Yeah, that’s a good point. I mean, we’ve all seen dashboards where if you often sometimes if you just leave it to technologists, you wind up getting the more data is better. Let me throw the kitchen sink as opposed to that hierarchical, what does the executive need to see?
What are the directors need to see? What are the ops people need to see? How do I segment this, etc?
I completely agree. I mean, that’s a huge underrated often component. And we all know it’s pretty rare that we see dashboards that are thought through from a UX perspective, exceptionally well, at least in our experience.
So let’s kind of hop back to we’re talking about dashboards. So what were the results and insights that ultimately, you guys were able to present to the client? And also act on ourselves, we’ll talk about that afterwards.
But the results and insights you’re able to see and present to the client through the data business that resulted from the work you did?
Ashley
Yeah, so one of the big insights, and because they’re running so many different campaign types, and we’re looking at a lot of different conversion metrics, like one of them, they’re really looking at churn and trying to mitigate that churn. So we know how many people churn, do people churn more if they interact with emails or less. So what we found was that we’re able to send more proactive messaging based around that churn, where how do we keep people from turning before it happens, and then able to measure the success of that.
So we sent the message before the end of a season. And we’re able to tell that that messaging really helped to reduce reduce some of that churn. So that was a huge one.
Another one that we have for, I think, engagement, where we’re looking at do users who engage with email more have a higher lifetime value than users who either engage less or don’t engage at all. So what we found was that users who do engage in those different groups of high, low, medium, that the more people engage, the higher that we found their lifetime value was. So when it comes to activation, of course, when we pull this data into a CDP, we of course, are really wanting to message to these users differently.
If we have high engaged users, and we know their lifetime value is higher, we want to get those low engaged users to the medium and the medium to the high. So of course, you’re going to message to them differently. Whereas currently, or prior to that, all of those users, current subscribers were in one bucket.
Whereas now we can really be a lot more specific in that messaging to them to try and get them into those higher engagement categories.
Aric
Got it. And I know, Jack, I mean, obviously, with what Ashley is saying there, then become after you do that, that first layer, there come the questions of causality. So meaning, do people who are already not going to churn, therefore engage at a higher level with email, or if we can get them to engage with email more, will that reduce their churn?
So I know those sorts of analyses are on the roadmap now that you built out this infrastructure, right?
Jack
Yeah, oh, yeah. We’re doing a lot of really great things, collaborating with our data scientists on segmenting these users, deriving prediction scores, such as prediction, cancellation prediction for our churn mitigation. So that’s a big thing that’s on the roadmap, coming very soon, where we are kind of tracking that user level prediction score over time, layered in with these intervention measures that we’re launching.
And then we’ll be able to measure the effectiveness of those campaigns by looking at that cancellation score over time for those users to say, hey, this user, or this email, or this, you know, touchpoint went out to these users, at this point in time, these are the users that engaged, did we see a reduction in their cancellation prediction to measure the success of those campaigns? So it’s not even really a conversion metric, which is, again, why it’s so important for us to get this stuff out of the platform and into our own environment to measure more complex things like that. But yeah, that was a big collaboration with our data scientists for segmenting those users so that we could activate through their CDP, effectively, you know, from their CDP into their lifecycle marketing tool by these different cohorts.
Aric
I know both of you have mentioned CDPs, customer data platforms, for anyone listening that doesn’t know the term, talk more about the connection there, because that’s really a powerful capability and evolution of this project.
Jack
Yeah, so their CDP is layered on top of the data warehouse, as well as through all the different platforms that they engage with consumers on. But by fueling the CDP with all of this data from the data warehouse allows us to create really advanced audiences in the CDP, or in the data warehouse, sending that into the CDP, and then using the CDP to facilitate those campaigns into lifecycle marketing, into social media, into search and other platforms in a way that, hey, we want to go after these medium engagement users and get them up to a highly engaged user. We can create that audience in the data warehouse and then into the CDP, where that audience can be sent to all those different channels, so that those users are seeing the same type of messaging across all of those platforms, because that CDP, you know, one audience to many different channels.
Aric
Got it. Got it. Well, really interesting evolution there.
So we’re almost up on time. Ashley, Jack, anything else you might want to add about the project or the process or anything like that?
Jack
No, this was a really fun one. A really exciting opportunity for us to collaborate cross-functionally across our organization and bring a lot of folks together to develop something that provided a lot of value to the client, and best of all, in a very measurable way, because we were able to see the lift that was created based off of the decisions made from the data visualizations, you know, from all of that data that we all kind of brought together.
Aric
Yeah. I mean, it is really exciting. And I know that, I mean, our work with this client has been going on about three years, all the way from when we built the original data infrastructure from the ground up for the client.
But it’s also noteworthy. I really want to give you guys and the rest of the Center of Excellence credit that it’s made such differences in client media spend as well, how they should allocate, how much should be focused on acquisition versus retention, et cetera. And the cancellation prediction has come into that, right, to focus on, we need to put some media dollars toward retention efforts, et cetera, and understanding who and how much and when and what, et cetera.
So, it’s had really strong downstream impacts for this client and other clients that we do this kind of work for. So, again, thanks to you both for taking time out of the busy day to do this. And thanks to everyone in the Center of Excellence that’s not on this webinar.
But I guess we will wrap it up there and look forward to future webinars and future projects that you all can talk about.